Introduction to AI in Clinical Trials
The advent of Artificial Intelligence (AI) in healthcare has ushered in a new era of data-driven decision-making that holds the promise of revolutionizing clinical trial design and recruitment. AI, which encompasses machine learning (ML), deep learning (DL), natural language processing (NLP), and other complex computational techniques, is being rapidly integrated into the clinical research domain to streamline trial processes, reduce costs, and enhance patient outcomes. In clinical trials, AI is used not only to optimize study protocols and predict clinical outcomes but also to improve participant identification, engagement, and retention—addressing longstanding challenges in trial planning and execution. As clinical trials continue to be the backbone of drug and device regulatory approval, the integration of AI can radically transform how these trials are conceptualized, structured, and monitored.
Definition and Basic Concepts
Artificial Intelligence in clinical trials refers to the use of advanced computational algorithms and models to mimic human cognitive processes and provide insights based on large, complex datasets. Through machine learning techniques, AI can detect patterns and predictive signals from historical clinical data, thereby enabling researchers to simulate trial outcomes and refine their protocols before launching actual studies. Basic concepts of AI in this context include:
Machine Learning and Deep Learning: Algorithms that are trained on historical clinical trial datasets to identify correlations between patient characteristics and treatment outcomes, thus allowing for the prediction of clinical trial success rates and the estimation of necessary sample sizes. Natural Language Processing (NLP): This technology is employed to process and transform unstructured text data contained in clinical trial protocols, patient medical records, and investigator notes into structured, machine-interpretable formats that can be used to enhance trial design, screening, and eligibility determination. Predictive Modeling: AI uses statistical and computational models to forecast clinical trial outcomes (e.g., survival rates, response to therapy) based on input variables such as patient demographics, disease biomarkers, and treatment histories.
By leveraging these technologies, AI promises a paradigm shift in how researchers approach the design and conduct of clinical trials by transforming a traditionally resource-heavy, manual process into a more precise, efficient, and data-driven operation.
Current Challenges in Clinical Trial Design and Recruitment
Despite the critical role clinical trials play in advancing medical knowledge, several persistent challenges are inherent in traditional trial processes. These challenges have motivated the exploration of AI-driven alternatives.
Complex and Rigid Protocol Designs: Traditional clinical trial protocols are often based on static assumptions and heuristics derived from limited data. These designs may not reflect the inherent diversity and complexity of patient populations or evolving standards of care, leading to suboptimal inclusion and exclusion criteria. Consequently, many trials fail to enroll a sufficiently diversified cohort, compromising the robustness of the findings. Inefficient Recruitment and Enrollment: Slow patient recruitment is a well-documented bottleneck in clinical trials, frequently resulting in delays, increased costs, and even premature trial termination. Under-enrollment—often due to reliance on conventional recruitment methods such as physician referrals and traditional advertising—impacts not only the statistical power of the study but also the generalizability of its outcomes. This inefficiency can be exacerbated by geographical disparities and the lack of engagement with underrepresented populations. High Financial and Resource Burden: The clinical trial process is notoriously expensive, with trials in later phases consuming billions of dollars in investment. High failure rates due to misaligned patient selection and poor monitoring further compound these costs. The inability to dynamically adjust trial parameters as early signals of inefficacy emerge represents another significant challenge. Data Quality and Integration Issues: Clinical trials often rely on data that is siloed, incomplete, or unstructured, which hampers effective decision-making. The integration of disparate data sources—such as electronic health records (EHRs), imaging data, and behavioral data—into a coherent framework is a substantial hurdle. Regulatory and Ethical Concerns: With the introduction of AI into clinical research, ethical and regulatory issues have gained prominence. Concerns about data privacy, algorithmic bias, and the transparency of AI's “black-box” decision-making processes need to be addressed before widespread adoption.
These challenges underscore the need for a more dynamic, data-driven, and patient-centric approach to clinical trial design and recruitment—one that AI is particularly well-suited to provide.
AI Applications in Clinical Trial Design
AI applications in clinical trial design aim to address many of the challenges outlined above by optimizing trial protocols and predicting outcomes, thereby improving both efficiency and efficacy.
Optimizing Trial Protocols
AI can transform trial protocol design by enabling dynamic, real-time adjustments based on comprehensive historical data analysis and predictive analytics.
Data-Driven Protocol Customization: AI leverages large-scale datasets comprising patient demographics, disease characteristics, and prior trial outcomes to generate evidence-based adjustments to protocol inclusion and exclusion criteria. This ensures that the patient population is representative and that the study is designed to detect clinically relevant outcomes. For instance, by analyzing electronic health records, AI can recommend adjustments to eligibility criteria that double the potential recruitment pool without compromising efficacy, thereby directly reducing trial attrition rates. Simulation and In Silico Trials: Advanced machine learning models can simulate entire trials using virtual patient cohorts (i.e., in silico trials). These simulations allow researchers to test various trial design scenarios, estimate statistical power, and evaluate the effects of modifying protocol parameters such as treatment dosages or regimen durations before actual patient enrollment begins. In silico trials are particularly effective at predicting clinical trial outcomes and identifying potential shortcomings in trial design. Dynamic Protocol Adaptation: Hybrid systems that integrate human input with AI-powered natural language processing are capable of dynamically structuring trial protocols from unstructured trial documents. These systems generate machine-interpretable trial designs that can automatically flag potential issues and optimize the protocol based on iterative learning from previously recorded trial data. This not only minimizes human error but also refines the design for future trials by incorporating lessons learned from past experiences. Reducing Bias in Design: AI-based optimization can help to reduce biases inherent in traditional trial designs. By integrating comprehensive patient data, AI systems can identify underrepresented groups and adjust eligibility criteria accordingly, ensuring fairer recruitment and more generalizable results. This approach is supported by AI’s ability to provide insights that human designers might overlook due to limitations in cognitive capacity or exposure.
Through these systems, AI can yield more flexible and robust trial protocols that are tailored to specific patient populations and real-world conditions. Researchers have noted that these improvements in trial design can lead to a reduction in overall trial duration and cost, while increasing the likelihood of detecting a true treatment effect.
Predictive Modeling for Outcomes
Predictive models powered by AI offer a transformative approach to forecasting clinical trial outcomes by analyzing complex, high-dimensional data.
Risk Stratification and Outcome Prediction: Machine learning models, especially those employing deep learning techniques, can predict patient-specific outcomes by identifying patterns related to survival, drug response, and adverse events. For example, approaches like Trial Pathfinder have employed historical electronic health record data to simulate trial outcomes and assess the impact of different eligibility criteria modifications on survival rates. Such predictive insights allow sponsors to optimize both trial design and patient stratification, ensuring that the trial not only recruits the right therapeutic candidates but also minimizes the risk of failure. Adaptive Trial Designs: AI enables the implementation of adaptive trial designs where ongoing results are continuously evaluated, and protocols are adjusted in real-time. These systems can modify sample sizes, treatment allocation ratios, and early-stopping rules based on interim analyses. Since only a fraction of compounds initially evaluated eventually make it through all phases of clinical trials, the ability to adaptively respond to patient responses and interim data can substantially increase trial success ratios. Learning from Real-World Evidence: Beyond controlled trial settings, AI tools can integrate real-world evidence—from registries, claims data, and EHRs—to improve the accuracy of predictive models. This integration helps in modeling real-life treatment effects more accurately, thereby overcoming some of the limitations of traditional trial designs that rely solely on controlled environments. Reduction in Sample Size Requirements: By providing more accurate predictions on treatment responses and outcomes, AI can help to design trials that require fewer patients while still maintaining statistical power. This reduction in sample size is crucial, as more efficient trials imply lower costs, shorter recruitment periods, and a faster path from development to regulatory approval.
AI-powered predictive modeling thus offers a means to not only optimize trial protocols but also to forecast their outcomes with greater precision. This ensures that potential opportunities and pitfalls are identified early in the trial process, ultimately leading to better resource allocation and increased trial efficiency.
AI in Recruitment Strategies
One of the most significant hurdles in clinical research is effective recruitment. AI can leverage big data and predictive analytics to streamline participant identification, engagement, and retention—thus addressing this fundamental challenge.
Enhancing Participant Identification
AI brings innovation to the participant identification process through its capability to analyze and interpret vast volumes of data.
Utilization of Electronic Health Records (EHRs): AI systems combine data mining and NLP to extract relevant patient information from EHRs. By doing so, they identify individuals who meet specific eligibility criteria for clinical trials. This approach, often coupled with HIPAA-compliant de-identification algorithms, ensures that patient privacy is protected while still enabling effective screening. For example, studies have shown that AI can rapidly scan large datasets to flag potential participants based on laboratory results, imaging data, and demographic characteristics. Real-time Data Integration: AI algorithms can integrate multiple data sources—including medical records, genomic data, and even social media profiles—to build comprehensive patient profiles. This multidimensional view of patient data allows for more precise matching of patients to trials based on both clinical and lifestyle factors. Such precision not only enhances the diversity of the participant pool but also ensures a better fit between the trial requirements and the patient’s health status. Predictive Candidate Screening: Techniques involving machine learning models – such as Random Forest, XGBoost, and deep neural networks – are employed to predict patient eligibility by analyzing historical trial enrollment data. These models calculate probabilities of patient match efficacy and, using probabilistic models, they rank potential candidates based on the likelihood that they will meet trial criteria and complete the study. This results in more efficient screening and matchmaking processes, minimizing wasted resources. Automated Clinical Trial Matching Systems: Several AI-powered platforms have been developed to automatically match patients with ongoing clinical trials. By leveraging both structured data (clinical parameters) and unstructured data (narrative text from physician notes), these systems can provide a continuously updated list of potentially eligible participants. Such platforms reduce the manual burden on clinical staff and improve the speed and accuracy of the recruitment process.
The capability of AI to integrate and analyze diverse data sources makes it a powerful tool for improving participant identification. By automating screening and matching processes, AI not only accelerates recruitment but also enhances the representativeness and diversity of enrolled populations.
Improving Engagement and Retention
Beyond identifying the right participants, AI can also play a vital role in keeping subjects engaged throughout the trial process, thereby reducing dropout rates and ensuring data integrity.
Personalized Communication Strategies: AI-powered chatbots and virtual assistants are capable of personalized interactions with potential and enrolled participants. These systems use NLP to comprehend patient concerns and provide tailored responses, appointment reminders, or educational materials—even in multiple languages—to cater to diverse patient groups. This personalized engagement ensures participants feel heard and supported, which in turn improves adherence and reduces dropout rates. Remote Monitoring and Digital Biomarkers: Wearable devices and mobile health applications integrated with AI algorithms enable continuous real-time monitoring of patient health during the trial. AI interprets data streams from these devices to detect deviations or adverse events promptly, thereby alerting healthcare providers to intervene before the patient’s condition deteriorates. Continuous monitoring not only improves patient safety but also gives participants confidence in the study process, enhancing retention. Adaptive Engagement Based on Feedback: AI allows real-time adjustment of engagement strategies based on patient feedback and behavior. For example, if a patient is less active in responding to digital prompts, the system can automatically switch to a more engaging format (such as a video message) or escalate to human support intervention. This adaptive framework minimizes patient burden and increases overall trial satisfaction. Minimizing Administrative Burden on Clinicians: By automating routine tasks such as scheduling, follow-ups, and data entry, AI liberates clinical staff to focus on more personalized patient interactions. When clinicians have more time to directly interact with patients, it builds trust and satisfaction, indirectly boosting retention rates. Research has indicated that improved clinician–patient interactions are correlated with higher retention rates in clinical trials. Enhanced User Interfaces and Dynamic Displays: AI-driven systems often incorporate dynamic interfaces to display trial-relevant information that adjusts as participants provide more responses. This iterative process helps maintain participant interest and reduces the cognitive load associated with traditional trial questionnaires. Such systems are designed to minimize question burden while still collecting all necessary data—a critical factor in maintaining long-term engagement.
By deploying these engagement strategies, AI ensures ongoing communication and continuity in patient care throughout the trial period. This comprehensive approach not only improves clinical outcomes but also significantly reduces the dropout rates that often plague traditional clinical trials.
Ethical and Regulatory Considerations
As AI gets more embedded in clinical trial design and recruitment, ethical and regulatory considerations become increasingly important. These considerations ensure that the tremendous potential of AI is harnessed in ways that are both responsible and compliant with legal standards.
Ethical Implications of AI in Trials
Integrating AI into clinical research brings forth several ethical challenges that must be addressed to build trust among stakeholders and ensure safe implementation.
Data Privacy and Confidentiality: AI systems rely on enormous datasets, often containing sensitive personal health information. Ensuring that this data is de-identified and stored securely is essential to maintain patient privacy. Strict adherence to regulations such as HIPAA is imperative, and AI systems must be designed with robust encryption and privacy safeguards. Algorithmic Bias and Fairness: One of the most pressing ethical concerns is the potential for AI systems to perpetuate or even exacerbate biases present in the historical data they are trained on. Bias in patient selection or treatment response predictions can lead to inequitable outcomes, particularly affecting minority or underrepresented populations. It is crucial to develop AI approaches that are continuously audited for fairness and that include diverse datasets during training. Transparency and Explainability: Many AI models function as “black boxes,” making it difficult for clinicians and patients to understand how decisions are made. The need for explainable AI (XAI) has therefore become a priority, ensuring that the reasoning behind trial design adjustments or patient matching is transparent and can be critically evaluated by human experts. Informed Consent and Patient Autonomy: When AI tools are used to influence clinical trial participation or treatment decisions, it is vital that patients are adequately informed about the role these tools play. Transparent disclosure of AI’s involvement helps to uphold patient autonomy and ensures that consent is based on complete information. Responsibility and Accountability: If errors occur due to AI-driven decisions, there must be a clear framework of accountability. Determining whether human clinicians or the AI developers bear responsibility is essential for maintaining ethical standards in clinical trial conduct.
Addressing these ethical implications is a fundamental step towards ensuring that AI enhances rather than undermines the integrity and fairness of clinical trials.
Regulatory Guidelines and Compliance
The successful integration of AI in clinical trials depends not only on technological advances but also on compliance with evolving regulatory frameworks. Regulatory bodies around the world are beginning to develop guidelines specifically for AI applications in healthcare.
Standardization of Protocols: Regulatory agencies such as the FDA and EMA are increasingly focused on ensuring that AI algorithms used in clinical research meet rigorous standards for accuracy, reliability, and transparency. This includes requirements for pre-market validation, post-market surveillance, and continual performance monitoring. Data Governance and Security Standards: Compliance with stringent data protection frameworks like HIPAA in the United States and GDPR in Europe is mandatory. AI systems deployed in clinical settings must incorporate state-of-the-art security measures to protect patient data and ensure that any data sharing is done within the boundaries of legal regulations. Guidelines for Ethical AI Use: Emerging guidelines, such as those encapsulated in the SPIRIT-AI and CONSORT-AI extensions, aim to standardize the way AI interventions in clinical trials are reported and evaluated. These guidelines help researchers ensure that every stage—from protocol development to endpoint analysis—is conducted and documented ethically. Integration with Established Clinical Trial Processes: Regulators expect that AI-enhanced clinical trials remain compatible with established clinical trial infrastructures. This means that any AI tool must be integrated with existing EHR systems and clinical trial registries seamlessly, and that trial outcomes derived from AI recommendations must be interpretable within the current regulatory framework. Ongoing Audits and Post-Approval Monitoring: Even after an AI tool is approved for use in clinical trials, regulatory bodies require continuous auditing to ensure that it continues to perform as expected without introducing new risks. This involves both internal reviews by the trial sponsors and independent evaluations by regulatory agencies.
Thus, adherence to ethical and regulatory standards is crucial to ensuring that AI’s integration into clinical trial design and recruitment is not only innovative but also safe, equitable, and legally compliant.
Future Directions and Innovations
The landscape of AI in clinical trials is evolving at a rapid pace, and emerging innovations promise to further enhance the efficiency, adaptability, and robustness of the clinical research process.
Emerging AI Technologies in Clinical Trials
Researchers and industry stakeholders are actively exploring new AI technologies that could further transform clinical trial design and recruitment.
Advanced Natural Language Processing: Next-generation NLP models can further improve the structuring of unstructured text data—such as patient narratives, clinical notes, and trial protocols—into standardized formats. This will facilitate automated trial matching and real-time protocol adjustments without extensive human oversight. Digital Twin and In Silico Trials: The concept of digital twins—in which virtual replicas of patients are created—can enable simulated trials that allow researchers to test multiple scenarios simultaneously. These systems can dramatically reduce trial costs by optimizing treatment protocols before physical trials commence and by generating virtual control groups to supplement real-world data. Real-time Adaptive Recruitment Platforms: Emerging platforms that integrate wearable technologies, smartphones, and IoT devices with AI algorithms will allow for continuous monitoring of patients during trials. These systems will not only improve the accuracy of recruitment but also offer real-time feedback loops to adjust the recruitment strategy dynamically as the trial progresses. Explainable AI Models: Investment in research on explainable AI (XAI) will ensure that future systems provide transparent, understandable justifications for their recommendations regarding trial design and recruitment. This can help build trust among clinicians and patients and aid regulatory compliance in the long term. Integration of Multi-modal Data Analytics: Future AI systems will be able to combine imaging data, genetic profiles, EHRs, and even socio-economic data to predict trial outcomes more accurately. These multi-modal AI models can help in designing more patient-centric trials that are adaptive to the local specifics of patient populations. Blockchain Integration for Data Security: Blockchain technology combined with AI can provide enhanced data integrity and security, offering an immutable log of data access and modifications. This integration is likely to be critical for maintaining patient trust and regulatory compliance in AI-augmented clinical trials.
Long-term Impacts and Research Opportunities
The integration of AI into clinical trials is just the beginning. Long-term research and innovation in this domain are expected to yield transformative impacts on the overall clinical research landscape.
Reduction in Drug Development Timelines: With AI optimizing trial design and enhancing recruitment and retention, the overall timeline for drug development could be substantially reduced. Studies indicate that even modest improvements in trial efficiency can lead to savings of millions or even billions of dollars, accelerating the delivery of new treatments to the market. Personalized and Precision Medicine: AI’s ability to analyze patient-specific data in real-time lays the groundwork for highly personalized therapies. Future clinical trials might adopt adaptive designs that tailor treatment arms based on intermediate patient responses, fostering a more robust precision medicine approach. Improved Statistical Power with Smaller Cohorts: By optimizing inclusion criteria and employing sophisticated predictive models, AI can help design trials that require smaller cohorts while maintaining statistical validity. This allows trials in rare diseases or niche patient populations to be more viable and cost-effective. Enhanced Global Collaboration: The use of integrated AI systems can facilitate international multi-center trials by harmonizing data standards and reducing the barriers imposed by geographical variation. This can lead to more diverse and comprehensive datasets, ultimately resulting in more generalizable clinical trial outcomes. Continuous Learning and Improvement: One of the most promising long-term impacts will be seen in AI systems that continuously learn and update from every trial. This iterative improvement can transform clinical research into a continuously evolving field where each trial informs the next, leading to a self-improving ecosystem of clinical knowledge. Interdisciplinary Research Opportunities: The intersection of AI with clinical research opens vast opportunities for interdisciplinary collaborations among computer scientists, clinicians, ethicists, and regulatory experts. Such partnerships will be necessary to overcome current challenges and to develop innovative trial models that are both clinically effective and ethically sound. Transformative Impact on Health Care Systems: In the long run, AI has the potential not only to transform clinical trials but also to enhance overall healthcare delivery by enabling more efficient and patient-centered treatment pathways. The knowledge generated from AI-optimized trials can feed back into routine clinical practice, creating a virtuous cycle of improvement across the entire healthcare system.
Through these emerging AI technologies and long-term research trajectories, the future of clinical trials appears poised for radical transformation. The adoption of AI-centric frameworks is expected to foster a more innovative, cost-efficient, and patient-friendly clinical research environment.
Conclusion
In summary, AI has immense potential to improve clinical trial design and recruitment by addressing both longstanding and emerging challenges in the current clinical research ecosystem. On a general level, AI transforms clinical trial design into a dynamic, data-driven process that can optimize trial protocols, reduce sample size requirements, and enhance outcomes prediction. Specifically, through advanced data analytics, simulation models, and real-time adaptive trial designs, AI refines eligibility criteria and risk stratification—thereby improving trial specificity and efficiency.
From a recruitment perspective, AI facilitates the identification of eligible participants by integrating multi-modal datasets from EHRs, genomics, and other sources, and by deploying automated matching algorithms that streamline the screening process. AI-driven engagement tools, such as chatbots and remote monitoring via wearables, ensure ongoing patient involvement, thereby enhancing retention and reducing dropout rates. This dual improvement in trial design and recruitment can lead to significant cost reductions, faster trial completion times, and ultimately, more robust evidence for new treatments.
Equally important are the ethical and regulatory aspects. Ensuring data privacy, mitigating algorithmic bias, and improving transparency via explainable AI techniques are vital for building trust and maintaining compliance with evolving guidelines. Regulatory bodies are increasingly demanding standardized protocols for AI applications in clinical trials, and the emerging SPIRIT-AI and CONSORT-AI guidelines represent steps in that direction. These measures reassure stakeholders that the benefits of AI will not come at the expense of ethical standards or patient safety.
Looking forward, emerging technologies such as digital twins, enhanced multi-modal analytics, blockchain integration, and advanced NLP all promise to further enhance AI’s role in clinical research. Long-term impacts include more personalized medicine, shorter drug development timelines, improved global trial collaboration, and an evolving ecosystem where every trial contributes to a continuously improving model of clinical research. Collaborative efforts spanning multiple disciplines will be key to unlocking these opportunities and ensuring that AI’s integration benefits broader society while addressing inherent ethical challenges.
In conclusion, AI represents a transformative force in clinical trial design and recruitment. Its capabilities in protocol optimization, predictive modeling, and participant engagement are already producing favorable outcomes. By ensuring robust ethical frameworks and adhering to evolving regulatory guidelines, AI can accelerate the clinical trial process, thereby enhancing treatment development and ultimately improving patient outcomes. The future is promising, with ongoing research poised to further integrate AI technologies into all aspects of clinical research, heralding an era of more efficient, inclusive, and innovative healthcare solutions.
For an experience with the large-scale biopharmaceutical model Hiro-LS, please click here for a quick and free trial of its features!
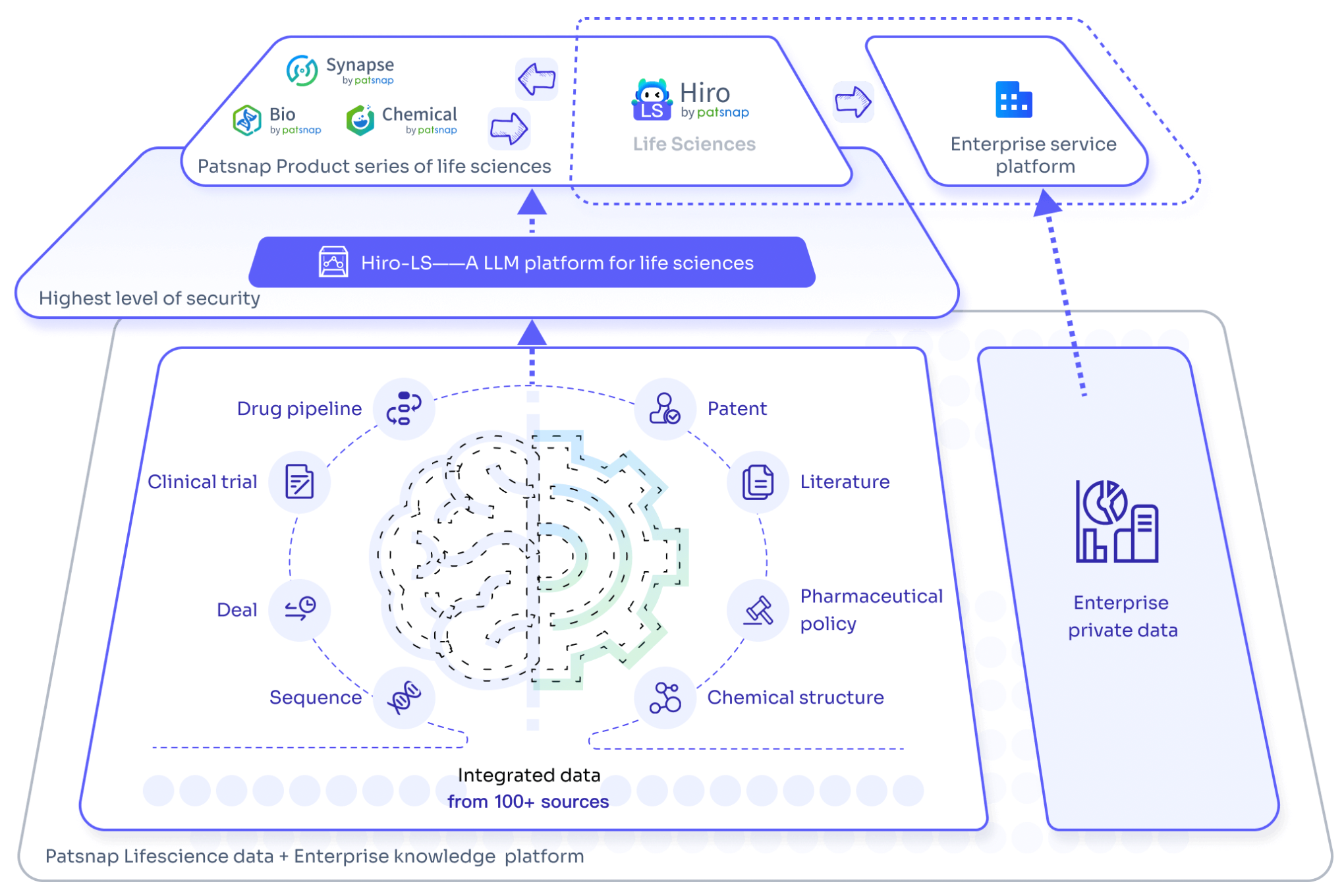